Detection and classification of cardiovascular diseases using neural networks
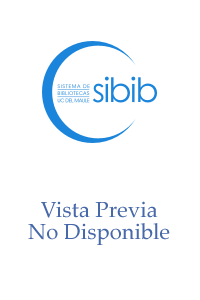
Autor
Estay Zamorano, Bastián
Dehghan Firoozabadi, Ali
Brutti, Alessio
Adasme, Pablo
Zabala-Blanco, David
Palacios Játiva, Pablo
Azurdia-Meza, Cesar A.
Fecha
2024Resumen
This research proposes the use of convolutional neural networks (CNNs) for classifying the electrocardiograms (ECGs) using continuous wavelet transform (CWT). Various CNN architectures were compared and optimized, which employ an algorithm that halts training when the network converges. Three PhysioNet databases were collected, temporally segmented, and transformed into scalograms using CWT. Training was conducted in MATLAB, initially with the stochastic gradient descent (SGD) optimizer with Momentum and secondly with adaptive moment estimation (ADAM) optimizer, to assess its impact on learning. The results indicate that both AlexNet and GoogleNet are the best networks in terms of accuracy and training time. ADAM improves performance and training time across all networks compared to SGD, and a decrease in performance was observed when the signals were affected by noise.
Fuente
Signal Processing - Algorithms, Architectures, Arrangements, and Applications Conference Proceedings, SPA, 2024, 132-137Link de Acceso
Click aquí para ver el documentoIdentificador DOI
doi.org/10.23919/SPA61993.2024.10715609Colecciones
La publicación tiene asociados los siguientes ficheros de licencia: