Analysis of spectrum detection and decision using machine learning algorithms in cognitive mobile radio networks
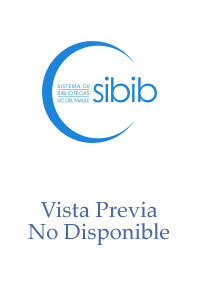
Autor
Palacios Játiva, Pablo
Azurdia-Meza, Cesar A.
Sánchez, Iván
Zabala-Blanco, David
Román Cañizares, Milton
Fecha
2021Resumen
In this work, the performance of four Machine Learning Algorithms (MLAs) applied to Cognitive Mobile Radio Networks (CMRNs) are analyzed. These algorithms are Coalition Game Theory (CGT), Naive Bayesian Classifier (NBC), Support Vector Machine (SVM), and Decision Trees (DT). The numerical results of the performance analysis of these algorithms are presented based on two metrics. These metrics are commonly used in CMRNs which are Probability of Detection (Pd) and Probability of False Alarm (Pfa) against Signal-to-Noise Ratio (SNR). Furthermore, outcomes regarding the Classification Quality (CQ) and the simulation time are exposed.
Theoretical and numerical results show that the SVM outperforms the rest of the algorithms in each of the metrics. The reasons behind this come from the SVM features, namely high precision, fast learning, and simplicity in the realization stage.
Fuente
Lecture Notes of the Institute for Computer Sciences, Social Informatics and Telecommunications Engineering, 381, 142-153Link de Acceso
Click aquí para ver el documentoIdentificador DOI
doi.org/10.1007/978-3-030-77569-8_11Colecciones
La publicación tiene asociados los siguientes ficheros de licencia: