Water status estimation of cherry trees using infrared thermal imagery coupled with supervised machine learning modeling
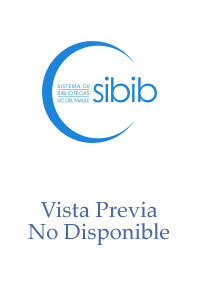
Autor
Gonzalez Viejo, Claudia
Tongson, E. J.
Baffico-Hernández, Antonella
Ávila Sánchez, Carlos
Fuentes, Sigfredo
Fecha
2022Resumen
The implementation of artificial intelligence (AI) in parallel with remote sensing could be a powerful tool to manage irrigation scheduling on crops with narrow thresholds between water stress levels, such as cherry trees. This research assessed the water status of 'Regina' cherry trees using machine learning (ML) modeling from data extracted automatically using infrared thermal imagery (IRTI). These models were used to predict stomatal conductance (gs) and stem water potential (Ψs) (Model 1) and a complete assessment using a matrix differential analysis procedure per IRTI of cherry tree canopies' temperature and relative humidity (Model 2). Results showed that the supervised ML regression models presented high and significant correlation coefficients (R = 0.83 and R = 0.81, respectively) without signs of overfitting assessed through their performance. The complex interactions among climatic factors, the soil moisture, and canopy architecture observed in cherry trees or any other fruit tree oblige exploring the performance of ML-based models to offer simple alternatives for decision-making processes in the field.
Fuente
Computers and Electronics in Agriculture, 200, 107256Link de Acceso
Click aquí para ver el documentoIdentificador DOI
doi.org/10.1016/j.compag.2022.107256Colecciones
La publicación tiene asociados los siguientes ficheros de licencia: