Raspberries-LITRP database: RGB images database for the industrial applications of red raspberries’ automatic quality estimation
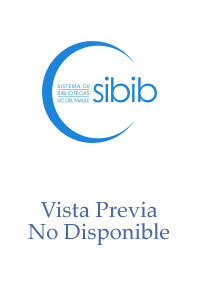
Fecha
2022Resumen
This work presents a free new database designed from a real industrial process to recognize,
identify, and classify the quality of the red raspberry accurately, automatically, and in real time.
Raspberry trays with recently harvested fresh fruit enter the industry’s selection and quality control
process to be categorized and subsequently their purchase price is determined. This selection is
carried out from a sample of a complete batch to evaluate the quality of the raspberry. This database
aims to solve one of the major problems in the industry: evaluating the largest amount of fruit possible
and not a single sample. This major dataset enables researchers in various disciplines to develop
practical machine-learning (ML) algorithms to improve red raspberry quality in the industry, by
identifying different diseases and defects in the fruit, and by overcoming limitations by increasing
the performance detection rate accuracy and reducing computation time. This database is made up of
two packages and can be downloaded free from the Laboratory of Technological Research in Pattern
Recognition repository at the Catholic University of the Maule. The RGB image package contains 286
raw original images with a resolution of 3948 × 2748 pixels from raspberry trays acquired during a
typical process in the industry. Furthermore, the labeled images are available with the annotations
for two diseases (86 albinism labels and 164 fungus rust labels) and two defects (115 over-ripeness
labels, and 244 peduncle labels). The MATLAB code package contains three well-known ML methodological approaches, which can be used to classify and detect the quality of red raspberries. Two
are statistical-based learning methods for feature extraction coupled with a conventional artificial
neural network (ANN) as a classifier and detector. The first method uses four predictive learning
from descriptive statistical measures, such as variance, standard deviation, mean, and median.
The second method uses three predictive learning from a statistical model based on the generalized extreme value distribution parameters, such as location, scale, and shape. The third ML
approach uses a convolution neural network based on a pre-trained fastest region approach (Faster
R-CNN) that extracts its features directly from images to classify and detect fruit quality. The classification performance metric was assessed in terms of true and false positive rates, and accuracy.
On average, for all types of raspberries studied, the following accuracies were achieved: Faster
R-CNN 91.2%, descriptive statistics 81%, and generalized extreme value 84.5%. These performance
metrics were compared to manual data annotations by industry quality control staff, accomplishing
the parameters and standards of agribusiness. This work shows promising results, which can shed a
new light on fruit quality standards methodologies in the industry.
Fuente
Applied Sciences, 12(22), 11586Link de Acceso
Click aquí para ver el documentoIdentificador DOI
doi.org/10.3390/app122211586Colecciones
La publicación tiene asociados los siguientes ficheros de licencia:
Excepto si se señala otra cosa, la licencia de la publicación se describe como Atribución-NoComercial-SinDerivadas 3.0 Chile
Publicaciones relacionadas
Mostrando publicaciones relacionadas por Título, autor o materia.
-
Establishment of photomixotrophic cultures for raspberry micropropagation in temporary immersion bioreactors (TIBs)
Arencibia-Rodríguez, Ariel; Vergara, Carolina; Quiroz, Karla; Carrasco, Basilio; García-González, Rolando (2013)
A novel protocol for raspberry (Rubus spp.) micropropagation in photomixotrophic conditions was optimized for the commercial genotypes Heritage, Meeker and Amity. Plant cultures were established in Temporary Immersion ...
-
Fruit-scan: system to automatically detect raspberry quality using computer vision techniques
Chile ranks tenth among the countries that export raspberries. In the Maule Region there are approximately 1,200 families who obtain their economic livelihood based on raspberry production. Raspberry exporting companies ...
-
Effects of four irrigation regimes on yield, fruit quality, plant water status, and water productivity in a furrow-irrigated red raspberry orchard
Ortega-Farias, Samuel; Espinoza-Meza, Sergio; Lopez-Olivari, Rafael; Araya-Alman, Miguel; Carrasco-Benavides, Marcos(2022)
Due to drought intensification in Mediterranean-type climates, raspberry growers need to implement irrigation scheduling to save water without decreases in yield and fruit quality. A study was performed to evaluate ...