Academic performance predicting model based on machine learning and keller's motivation measure
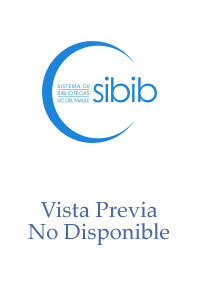
Autor
Laurens-Arredondo, Luis
Hernández-García, Ruber
Fecha
2022Resumen
This article investigates a model for predicting the academic performance of university students using Machine Learning techniques based on the level of motivation achieved with the implementation of the ARCS instructional model and the use of a technological tool called Arduino Science Journal used for learning Topics related to the kinematics of bodies. Analready validated methodology focused on motivation was implemented,which was quantified through the Instructional Material Motivational Survey (IMMS) instrument, which was applied toa group of 36 students of the Kinematics and Dynamics subject from a Civil Industrial Engineering career. Machine learning techniques were used to predict academic performance based on regression algorithms. The results show that Confidence was the IMMS dimension with the best prediction results. At the sametime, the Support Vector Regression algorithm achieves the lowest mean absolute error in the estimated academic performance. This research provides a prediction model of academic performance through emotional variables of the students, showing the potential to act as an early warning system, helping teachers to manage students' academic performance, and allowing students to self assess their performance.
Fuente
Proceedings - International Conference of the Chilean Computer Science Society, SCCC, 2022, 1-7Link de Acceso
Click aquí para ver el documentoIdentificador DOI
doi.org/10.1109/SCCC57464.2022.10000282Colecciones
La publicación tiene asociados los siguientes ficheros de licencia: