Machine learning-based classifiers to predict metastasis in colorectal cancer patients
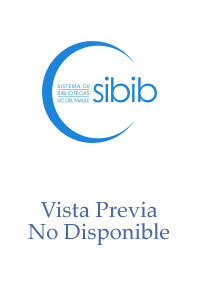
Autor
Talebi, Raheleh
Celis-Morales, Carlos
Akbari, Abolfazl
Talebi, Atefeh
Borumandnia, Nasrin
Pourhoseingholi, Mohamad Amin
Fecha
2024Resumen
Background: The increasing prevalence of colorectal cancer (CRC) in Iran over the past three decades has made it a key public health burden. This study aimed to predict metastasis in CRC patients using machine learning (ML) approaches in terms of demographic and clinical factors.
Methods: This study focuses on 1,127 CRC patients who underwent appropriate treatments at Taleghani Hospital, a tertiary care facility. The patients were divided into training and test datasets in an 80:20 ratio. Various ML methods, including Naive Bayes (NB), random rorest (RF), support vector machine (SVM), neural network (NN), decision tree (DT), and logistic regression (LR), were used for predicting metastasis in CRC patients. Model performance was evaluated using 5-fold cross-validation, reporting sensitivity, specificity, the area under the curve (AUC), and other indexes.
Results: Among the 1,127 patients, 183 (16%) had experienced metastasis. In the predictionof metastasis, both the NN and RF algorithms had the highest AUC, while SVM ranked third in both the original and balanced datasets. The NN and RF algorithms achieved the highest AUC (100%), sensitivity (100% and 100%, respectively), and accuracy (99.2% and 99.3%, respectively) on the balanced dataset, followed by the SVM with an AUC of 98.8%, a sensitivity of 97.5%, and an accuracy of 97%. Moreover, lower false negative rate (FNR), false positive rate (FPR), and higher negative predictive value (NPV) can be confirmed by these two methods. The results also showed that all methods exhibited good performance in the test datasets, and the balanced dataset improved the performance of most ML methods. The most important variables for predicting metastasis were the tumor stage, the number of involved lymph nodes, and the treatment type. In a separate analysis of patients with tumor stages I–III, it was identified that tumor grade, tumor size, and tumor stage are the most important features.
Conclusion: This study indicated that NN and RF were the best among ML-based approaches for predicting metastasis in CRC patients. Both the tumor stage and the number of involved lymph nodes were considered the most important features.
Fuente
Frontiers in Artificial Intelligence, 7, 1285037Link de Acceso
Click aquí para ver el documentoIdentificador DOI
doi.org/10.3389/frai.2024.1285037Colecciones
La publicación tiene asociados los siguientes ficheros de licencia: