Machine learning model for predicting primary school scores based on spatial, socio demographic and school–related information
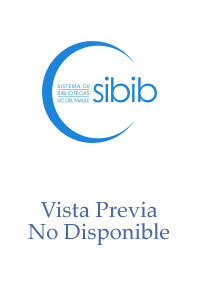
Autor
Lillo, Felipe
García, Leidy
Severino-González, Pedro
Fecha
2024Resumen
Learning strategies at primary school level are important to ensure student progress. In this regards, the identification of those factors influencing students grades certainly help teachers in predicting outcomes as well as in improving teaching strategies. This study empirically investigates connections between socio-demographic, school–related and academic features of primary students. Special attention is given to spatial features and how they influence performance. In particular the Euclidean distance from city center. The research method is based on machine learning techniques which are developed from a dataset consisting of 12159 primary school students living in the city of Talca, Chile. Four machine learning models are tested: a Neural Network (NN), Random Forest (RF) a Support Vector Machine (SVM) and a Gradient Boosted Tree model (GBT). Results show similar error levels between models and confirms student age, school capacity and school distance as important determinants of score predictions.
Fuente
Interciencia, 49(1), 60-67Link de Acceso
Click aquí para ver el documentoColecciones
La publicación tiene asociados los siguientes ficheros de licencia: