Multi-label classification to predict antibiotic resistance from raw clinical MALDI-TOF mass spectrometry data
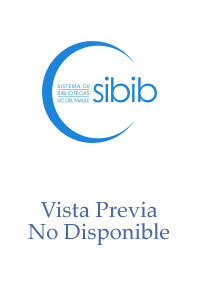
Autor
Astudillo, César A.
López-Cortés, Xaviera A.
Ocque, Elias
Manríquez-Troncoso, José M.
Fecha
2024Resumen
Antimicrobial resistance (AMR) poses a significant global health challenge, necessitating advanced predictive models to support clinical decision-making. In this study, we explore multi-label classification as a novel approach to predict antibiotic resistance across four clinically relevant bacteria: E. coli, S. aureus, K. pneumoniae, and P. aeruginosa. Using multiple datasets from the DRIAMS repository, we evaluated the performance of four algorithms – Multi-Layer Perceptron, Support Vector Classifier, Random Forest, and Extreme Gradient Boosting – under both single-label and multi-label frameworks. Our results demonstrate that the multi-label approach delivers competitive performance compared to traditional single-label models, with no statistically significant differences in most cases. The multi-label framework naturally captures the complex, interconnected nature of AMR data, reflecting real-world scenarios more accurately. We further validated the models on external datasets (DRIAMS B and C), confirming their generalizability and robustness. Additionally, we investigated the impact of oversampling techniques and provided a reproducible methodology for handling MALDI-TOF data, ensuring scalability for future studies. These findings underscore the potential of multi-label classification to enhance predictive accuracy in AMR research, offering valuable insights for developing diagnostic tools and guiding clinical interventions.
Fuente
Scientific Reports, 14, 31283Link de Acceso
Click aquí para ver el documentoIdentificador DOI
doi.org/10.1038/s41598-024-82697-wColecciones
La publicación tiene asociados los siguientes ficheros de licencia: